Continuing My AI Ethics Journey
- CGEST Staff
- Jun 28, 2022
- 5 min read
Updated: Jul 5, 2022
By Rachel Ware
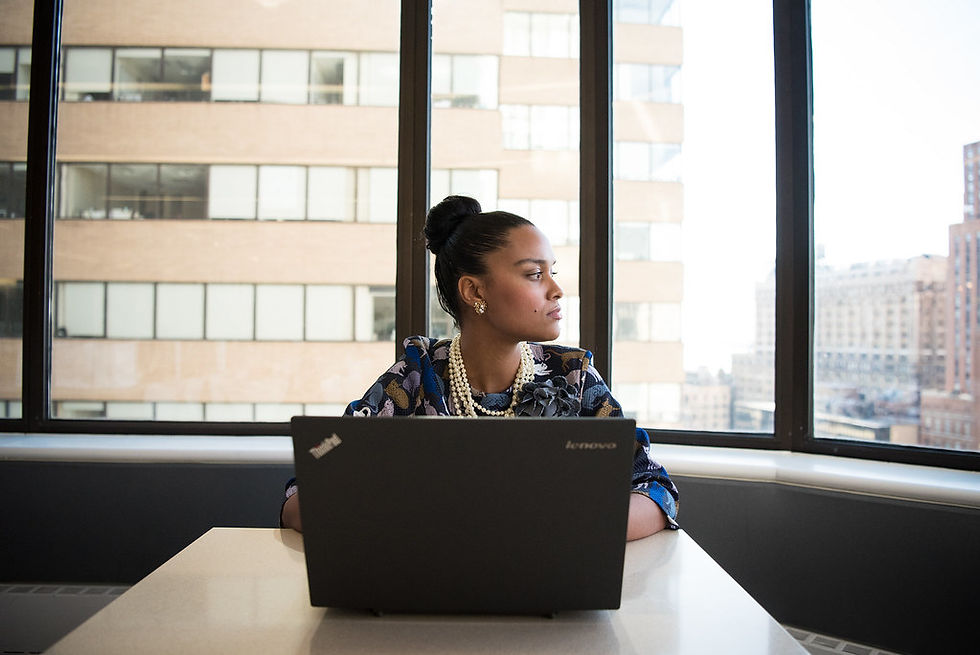
This past spring semester, I took a class about machine learning. It sparked a new interest in an area that was previously intimidating to me and provided me with much to think about. Despite getting comfortable with the type of coding I have had in my required classes (though those were also challenging), I had AI and ML as a sort of mythic space which I was worried about getting into. Everyone said the introduction class to AI was hard, and. I felt like I couldn’t get started until I had some more time to dedicate. But in the Fall, I took a class that had a guided final project on machine learning, and that gave me the push to try it. It was challenging. I finally learned why all that advanced math was required in my major and my knowledge was put to the test. I learned about how many types of machine learning exist and confirmed that it is, in fact, complicated.
In my Spring class, we had a group project where my group focused on transformers, which I wrote a bit about previously (link). During the presentations, another group had focused their project on bias and ethics in AI, which even from a short presentation pulled me in. This has been a topic I have heard about for a while, but it made me think more about the specific ways AI researchers address issues relating to bias and ethics. And it made me think about Timnit Gebru.
Timnit Gebru is a key figure in AI ethics research, and one of the co-founders of Black in AI (link), a non-profit which supports Black professionals and works to increase the presence of Black people in the field. She is also a co-author of Gender Shades, a paper that showed the biases in facial recognition technologies that were already in use. The technologies were less able to recognize people with darker skin and women (link). This paper was impactful not only to show the weaknesses but also to stop the use of these technologies in cases like policing. There was also the important point of the ethics of whether this technology should be used at all, given what it has been used for. I would really recommend reading the MIT Technology Review article linked above if you have not, and the original sources linked therein.
I had heard about this, and later in 2020, I heard about the news that made me aware of Timnit Gebru and look deeper. In December, I saw an article about the odd circumstances where an unpublished paper led to her being locked out of work accounts from where she worked in Google AI Ethics, and Google claiming she resigned (link). As a non-user of Twitter, I looked there anyway over the next few weeks to keep up with what was happening, because it was on my mind to follow tech companies’ approaches to work and try to understand the environment I would go into at different companies. It was upsetting to see that something like that could happen, and with so much support from AI Ethics professionals on Twitter, very worrisome about what that means for ethical oversight of AI. Re-reading now, the paper in this incident is talking about large natural language processing models - the same type of models I have just spent weeks learning about for my class project. These models get a lot of attention and positive reception, but in the article I found, they share that the paper talked about 4 major risks (link): environmental and financial costs, the huge amount of data (pulled from existing internet data), the research opportunity cost of continuing to focus on these models, and the use and misuse of models that look like human language (spreading misinformation or creating mistranslations). Some of these points were brought up in what I saw, but the focus was on the potential of the models, so re-examining this event, I am rethinking my previous understanding.
A big concern is in the types of biases that AI ethicists should be working on in the AI existing throughout society, and how much a researcher can do within the same company making and profiting on the technology’s use. How much incentive is there to prioritize ethics versus to look like you are? I don’t know enough of the specifics, but this is an area where it is easy to feel the technical aspects are so far beyond your reach that you can’t have an opinion. If companies say they are working on it but it is just too difficult, it must be. Or is there just not enough money going towards the research that would do that, and more towards the research that creates better-looking models which still do not understand what the language means that it uses. Models that use more energy and cost so much that only a few big companies can even work with them. As a current student, as someone who is learning more about AI, I feel a bit overwhelmed and like these sorts of issues are ignored in my introductions to ML. Like, shouldn’t this be more emphasized in classes? I was feeling unsure about the value of what I was learning, not in financial worth as that is brought up often, but in actual benefit to people beyond tech.
To engage with these thoughts, I wanted to check in again in 2022 and see where all this had gone. I got busy, and I focused on what was in front of me for a while, but now I learned some great news. In 2021, about a year after Google, Timnit started DAIR (link, link), the Distributed Artificial Intelligence Research Institute, one of many independent institutes considering these issues. They work from outside big tech companies to impact policy and shift the research or industry in ways that are not easy to do when beholden to a company (also mentioned in the article: Algorithmic Justice League, Data & Society, Data for Black Lives). Something I felt years ago when I first came to CGEST was the joy of shared language and vision of the future, and I see that when I look at their website. To quote: “We are an interdisciplinary and globally distributed AI research institute rooted in the belief that AI is not inevitable, its harms are preventable, and when its production and deployment include diverse perspectives and deliberate processes it can be beneficial. Our research reflects our lived experiences and centers our communities.”(DAIR website) and “However, we also believe that AI is not always the solution and should not be treated as an inevitability.” It is replenishing to read and hear these voices in this area. I feel it opens up my view of what is possible and where to look to be involved in the kind of technology that puts people and communities first. I personally look forward to what work they do and learning more about the people involved.
There is a lot of learning for me to do in machine learning, and I am currently in the process of understanding what aims or goals I have, but I wanted to share a bit of my journey because I am not sure it will be resolved, at least for a while. Even my idea for this post was much broader, but it is already long enough. There are heavy worries and excitement bundled up together, as I think there often are when examining the uphill of bias and inequity alongside learning about the amazing work being done to combat it. I hope to leave you a little more educated on the shifts in AI ethics, and ready to take it with a grain of salt when risks of AI are not presented to you.
References:
Image credit: WOCinTechChat.com
Comentários